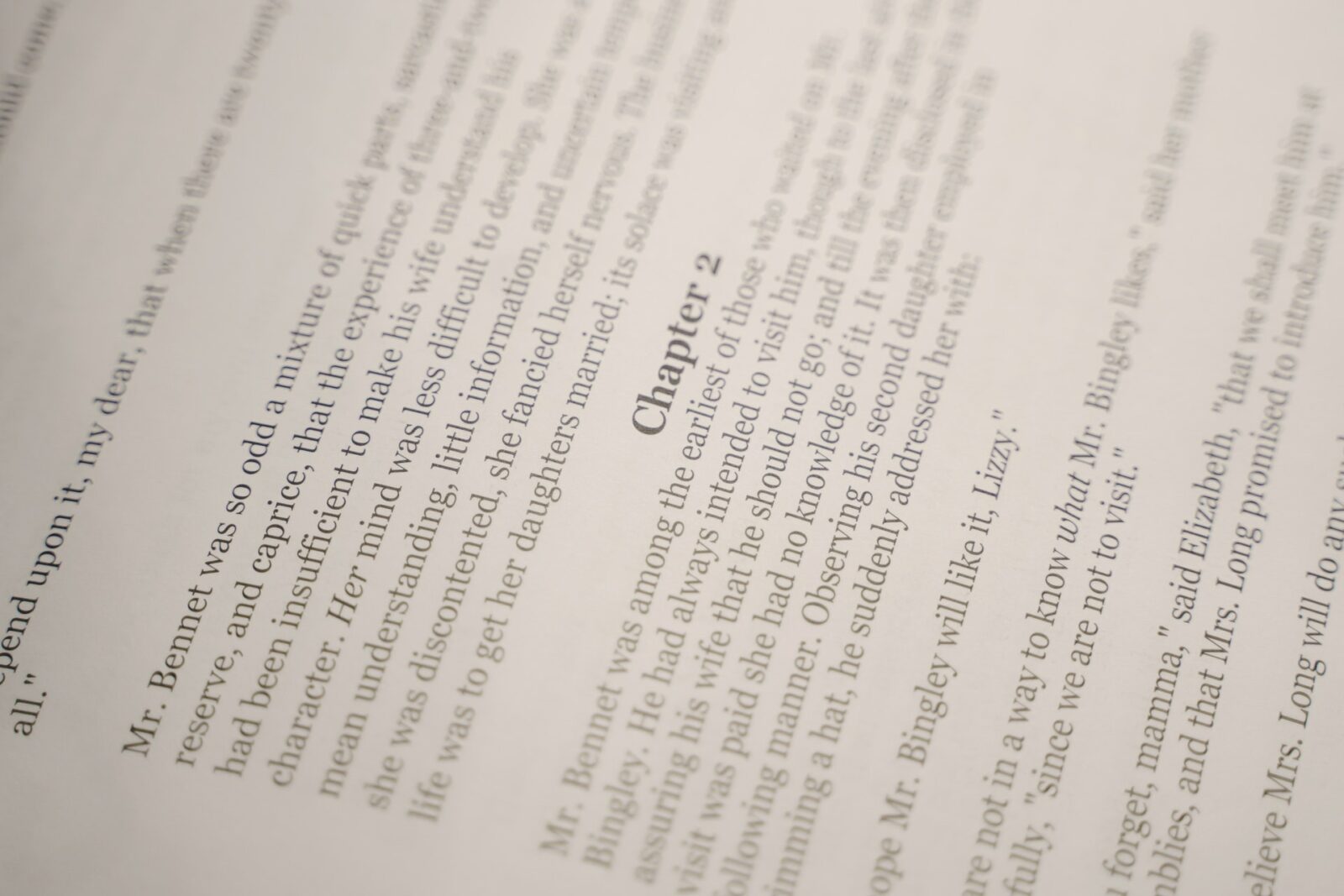
Transcribing interview and focus group content is tedious. Coding content in these interviews is when the hard work pays off. Put on your poet hat, because coding this content requires a keen eye for what words mean and how arrangements of words reveal meanings behind the words.
The images below are of a transcribed interview from research into music listening habits. When analyzing words and groups of words, develop codes that create “handles” for content. In the examples on this page, colors were used to clarify codes, with red indicating negative and green indicating positive. Here’s the process in action:
Page 6
- MU: Music type
- + : Positive feeling about something or a lot of something (combined with MU = positive feeling about a type of music)
- JAZZ : Jazz music
- COMF: Comfortable (one’s personal feeling of comfort)
- REB: rebeticko music
- SERBF: Serbian folk music
- NAME: Mention of a specific name of something or someone
- LIVE: Live music
- QUANT: A mention of a quantity that qualifies something (+QUANT = a lot)
- ACOUST: Acoustic music
- ELE: Electronic music
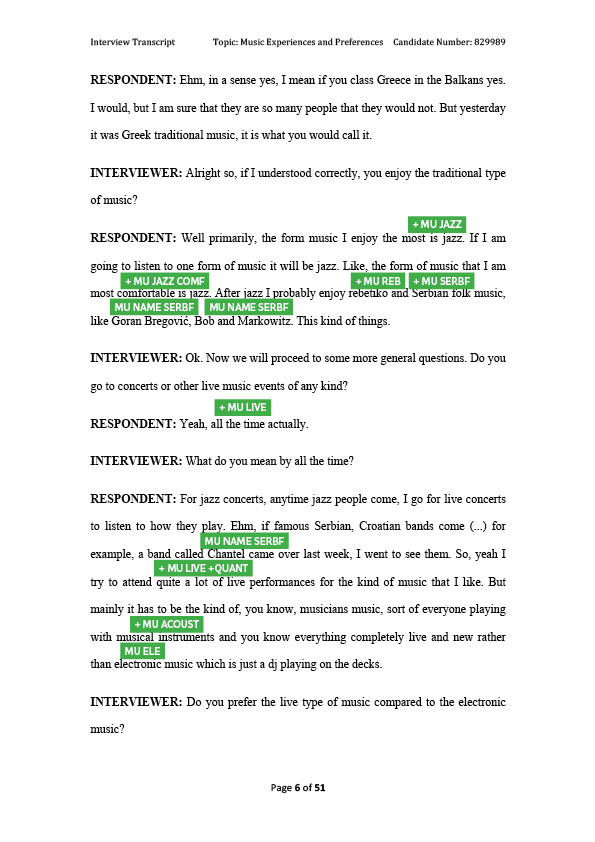
Page 18
- ALONE: being alone
- CHARSLOW: Character of music is slow
- CHARRMNTC: Character of music is romantic
- – : negative feeling about something
- LISTN: Mention of listening behavior
- POP: pop music
- SNG: Mention of a specific song
- CLASJAZZ: Classic Jazz music
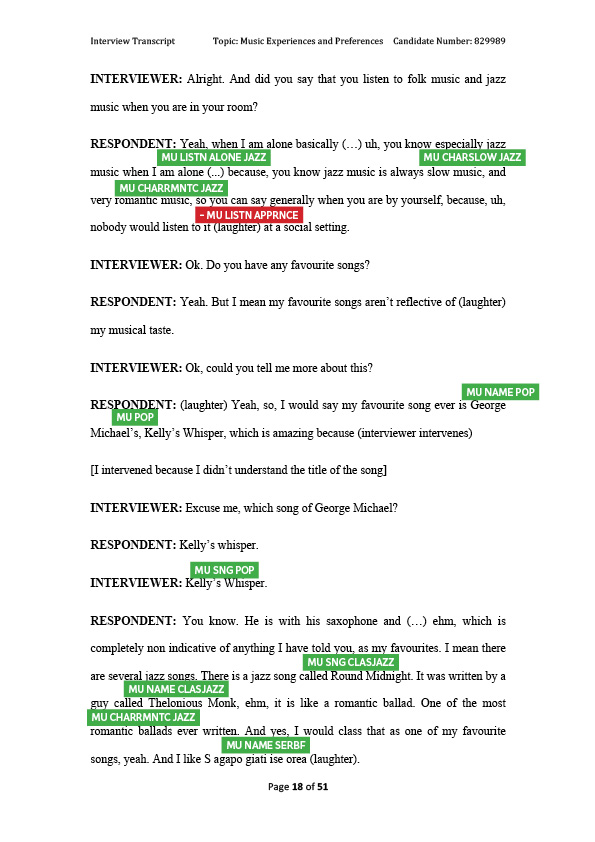
Page 39
- RAP: Rap music
- R&B: R&B music
- OPERA: Opera music
- ACTV: An activity
- PROG: Computer programming
- QUALREP: Repetitive quality of something
- CLEAN: Cleaning
- DANCE: Dancing
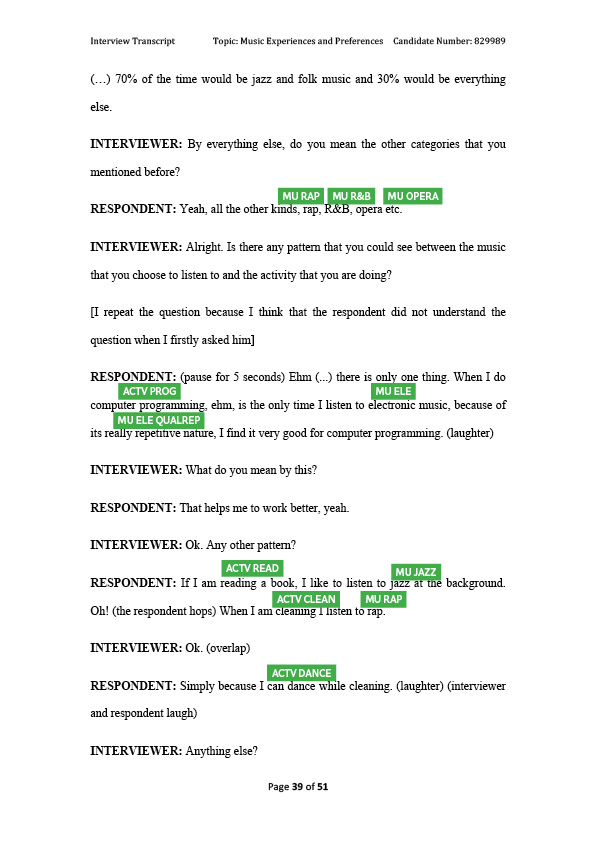
After 30 or so pages of interview transcription, a fairly clear picture of the participant’s music preferences should surface. Take that and multiply it by 5 or 10 participants, and themes should emerge that reveal a bit about the people whose behaviors are being studied.
Resources
Code interviews in small segments (Jazz; music, + and -, Activity, etc.) so each small code can be combined to create a clear picture that captures small nuances.
Pro Tip
Codes and trends don’t always reveal themselves on the first pass. Go through data more than once to affirm the codes you developed or determine if some were just outliers and should be ignored.